In 1966, renowned American engineer A. Michael Noll designed a computer program capable of creating semi-random geometric compositions in the style of Dutch artist Piet Mondrian, known for such abstract paintings as Composition with Red, Blue and Yellow and Broadway Boogie-Woogie, the latter of which is meant to represent the bustling streets of Manhattan as seen from atop a skyscraper. Mondrian’s work is often described as inimitable. But if this moniker holds true for human imitators, it does not apply to their mechanical counterparts. In fact, when Noll showed his program’s images to test subjects, only 28% of them could tell they weren’t made by an actual person.
In robotics, this kind of experiment is known as a Turing test. Named after British mathematician Alan Turing, who developed one of the first modern computers to decode encrypted German intelligence during the Second World War, the goal of these tests is to see whether or not a machine or software can pass off as human — and considering the rapid development of AI tools like ChatGPT and Dall-E, to name just a few, they will have to become a lot more rigorous if we want to distinguish between art and algorithm accurately.
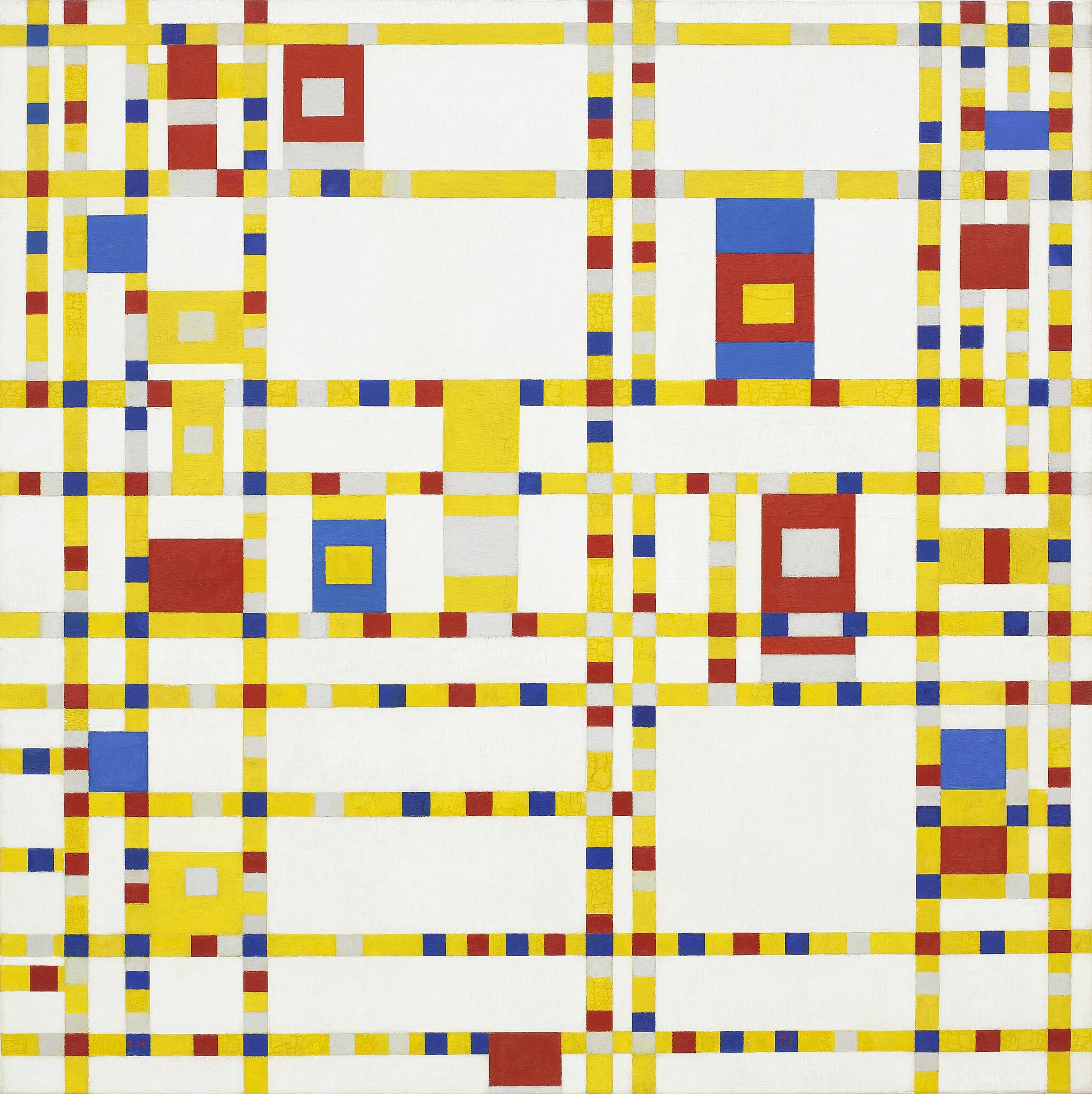
At least, that’s what Moscow-born artist and author Lev Manovich argues in Artificial Aesthetics: Generative AI, Art, and Visual Media. Written alongside philosopher Emanuele Arielli between 2021 and 2024, the book — publicly available online — not only explains how machine learning produces art on a technical level, but also teaches readers to recognize AI-generated images that a rudimentary Turing test would fail to unmask.
Rembrandt and Mondrian versus Duchamp
Not unlike professional artists, AI-powered text-to-image models create visuals based on what people find aesthetically pleasing. But whereas the former rely first and foremost on principles passed down through centuries of art history, the latter have access to a different, larger pool of data, including what types of styles and subjects attract the most attention on social media.
“Earlier predictions of image quality ratings were based on classical compositions, such as the rule of thirds, aspect ratio, saturation, and so on,” Manovich and Arielli write. Nowadays, neural networks go a step further, being able to “extract aesthetically relevant features through the analysis of large databases of liked images.”
Thanks to these advances, computer programs now pass the Turing test on a regular basis. Speaking to Big Think, Manovich points to the Dutch advertising executive Bas Korsten, whose algorithm — developed by data scientists from Microsoft and Delft University of Technology — managed to create a convincing portrait in the style of Rembrandt van Rijn after being trained on all 346 of the artist’s known paintings, complete with his trademark use of light and shadow, expressive brushstrokes, and moving facial expressions. In 2020, an undergraduate student from Princeton University accomplished the same thing, but with traditional Chinese landscape painting.
But AI is not just passing Turing tests for visual art. DeepBach, a neural network developed by Sony Computer Science Laboratories in Paris, can compose choral cantatas that music historians deem nearly identical to the music of Johann Sebastian Bach. Creative writing is also being conquered by AI. About a Hero, a murder mystery documentary that premiered this fall, was trained on the oeuvre of German filmmaker Werner Herzog, and is being met with rave reviews, despite Herzog’s own skepticism.
As impressive as these cases sound, Manovich insists their implications should be taken with a grain of salt. Limiting himself to the realm of visual art, he points out that some artistic styles are easier for AI to imitate than others. While a human painter requires years if not decades of training before they can produce paintings as lifelike as those by Rembrandt or Leonardo da Vinci, machine learning can achieve the same level of technical mastery in a matter of seconds. Not because it’s easy but because it’s based on the kinds of rules and patterns that machine learning excels at identifying and applying.
“Clearly recognizable styles represent well-defined problems that can be reduced to computational tasks,” Manevich says. Interestingly, it’s the artists who don’t follow a clearly discernable set of rules or patterns, such as Marcel Duchamp or Andy Warhol, that AI struggles to imitate effectively. Again, not because their art is abstract — so is Mondrian’s, after all — but because their constantly evolving styles, use of mixed media, and cerebral concepts result “in ill-defined tasks that have no easy procedural solution.”
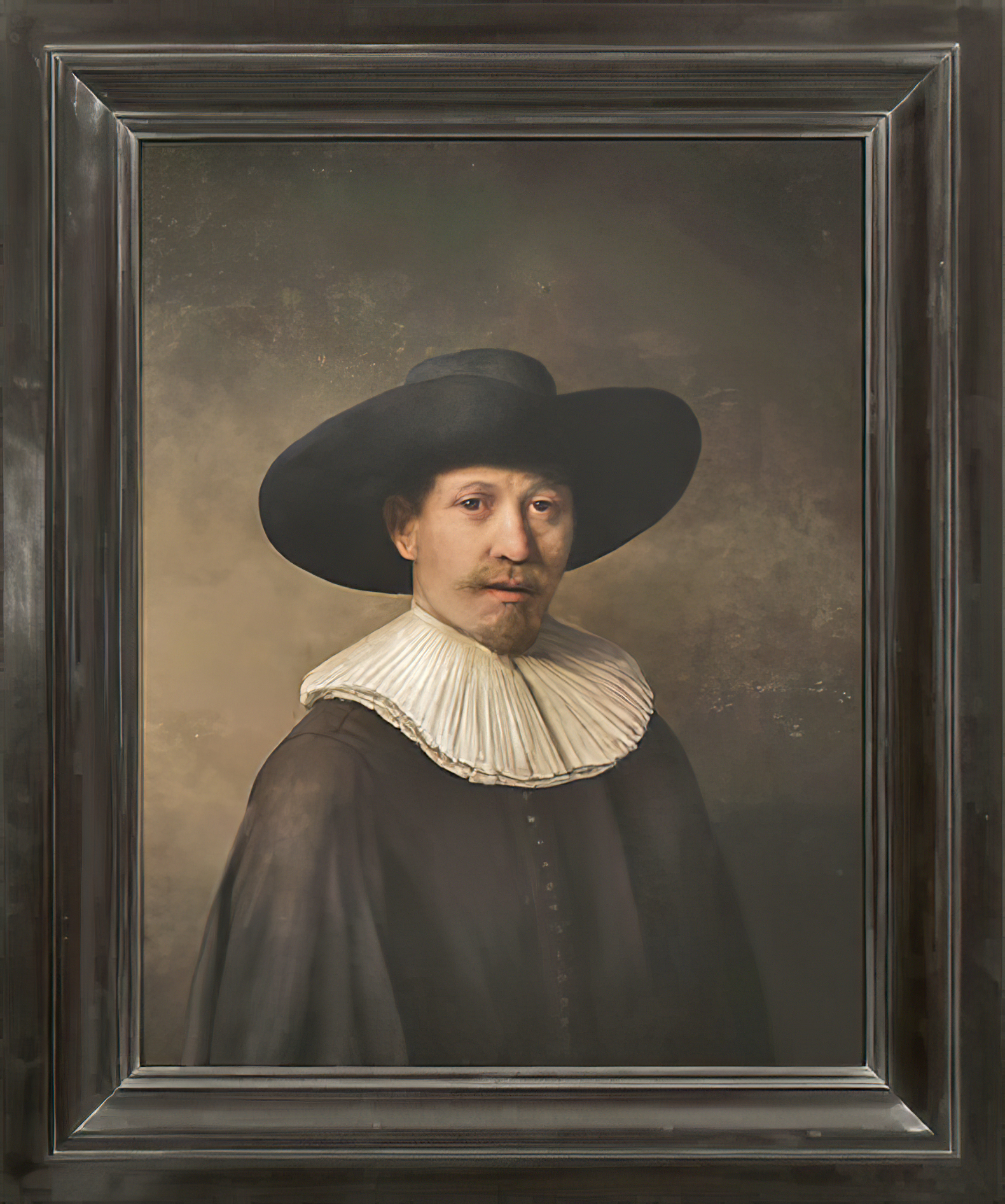
As Manevich and Arielli write in Artificial Aesthetics, “The popular cliché directed at contemporary art [namely, ‘My kid could have done that!’] seems now, in an ironic reversal, to turn against the great and stylistically complex — but computationally scalable — art of cultural tradition: even an AI could do that. It is the Duchamp that remains outside of AI’s creative abilities, at least for now.”
Turing testing
As skilled as AI-generated images have become at passing off for manmade art, there are a number of ways you can identify them. Aside from considering which art styles are easier to imitate than others, different generations of machine learning tools tend to have common aesthetics.
“We’re obviously talking about a moving target,” Manovich tells Big Think, “but historically, new media tends to have a recognizable look to it, like the early cinema from the late 1800s and early 1900s. Currently, a lot of AI-generated art features symmetrical compositions, with the object of interest smack in the center — quite different from what you’d find in classical or contemporary manmade art.”
Some other shared characteristics of AI-generated art include intricately rendered details, high resolution, and indiscernible, borderline surreal backgrounds — in short, precisely the sort of rules-based visual information that algorithms are best suited to come up with.
Less common in AI-generated art is visual information that isn’t necessarily rules-based, or based on rules that aren’t easily distilled into 1s and 0s, like the deliberate distortion of an object’s form and color to evoke a specific emotional response in the viewer, or the inclusion of a self-referential message or idea in the vein of Duchamp’s 1917 signed urinal, “Fountain,” which is not about the work itself so much as what it says about the history and culture of the art world.
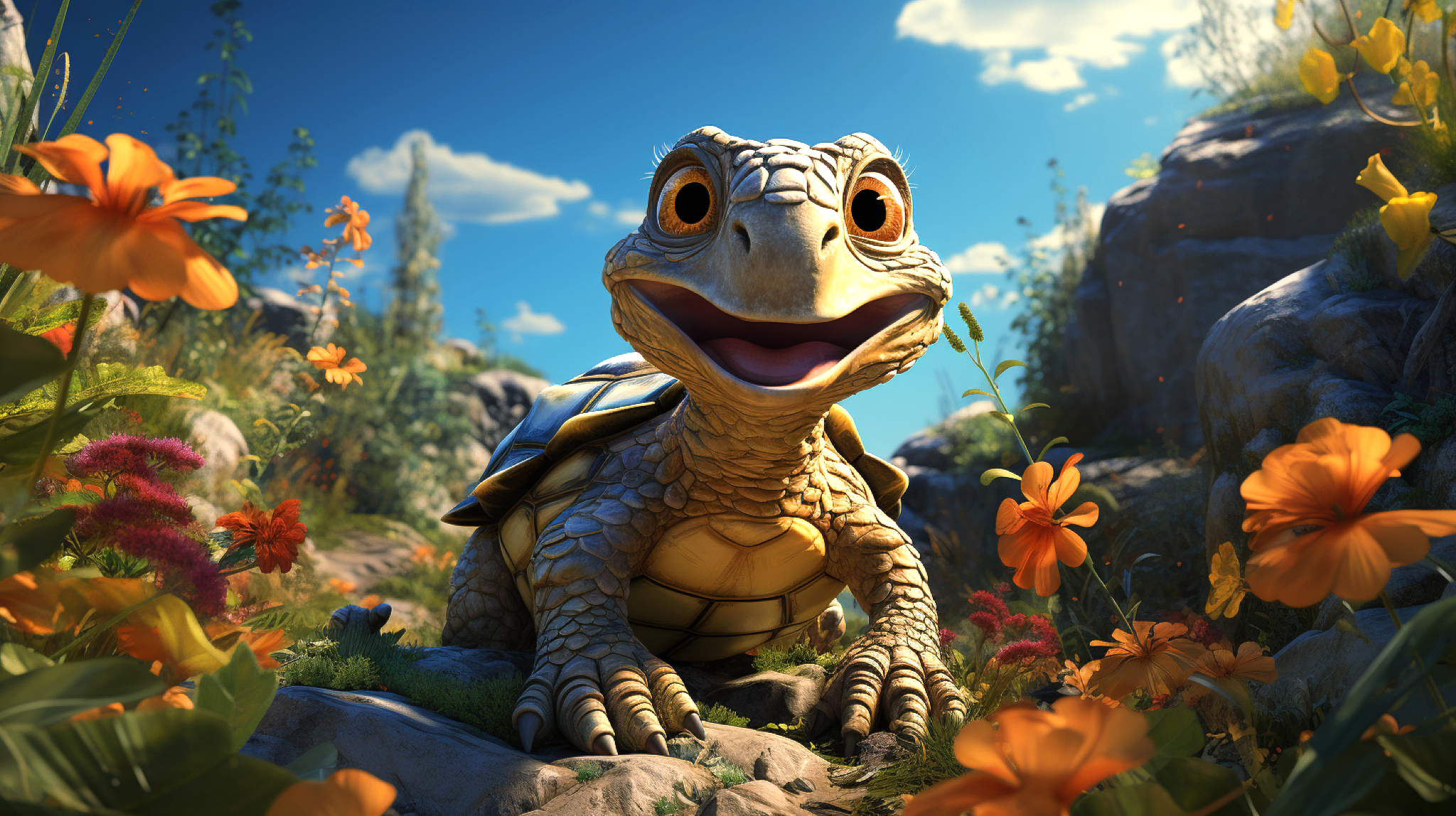
Aside from its practical applications, the ability to distinguish AI art from its human, real-deal counterpart via more rigorous Turing tests raises questions about the nature of creativity. Put simply: If people can’t tell whether a given image was generated by a person or program, what does this say about the value of a computer-generated image?
Manovich, for one, does not believe that AI heralds the end of art history. If anything, he believes the technology’s implications function as a logical continuation of art produced during the 20th century.
“In modern art,” he says, “artists often rejected the idea of exercising complete and total control over their work. They saw the machinery and materials they used as an integral part of their creative process. Andy Warhol, for instance, filmed the Empire State Building for hours, refusing to interfere with the footage. Others used automatic writing or random number generators. This tradition of using structures and systems fits with AI-generated art, which likewise challenges the notion that art is only valuable if created by a human being.”
“In fact,” he adds, “in the early days of digital art, artists used algorithms and random numbers, making their work perhaps even more ‘machine-like’ than today’s AI, which we control by selecting training data and crafting prompts. AI’s current form is more directed, meaning it could be seen as more of a collaborative tool than pure machine art.”
If artistic collaboration becomes humans and AI becomes ubiquitous, we might collectively choose to ditch the task of trying to discern who made a piece of art. It may simply be too tedious: “…the impossibility of true demarcation may lead to a “post-artificial” situation, […] where we ultimately suspend judgment on the true authorial origin of a work, permanently abandoning the question of whether something is genuinely “made by humans” or not.”
This article Why we need a better Turing test for AI art is featured on Big Think.

The post “Why we need a better Turing test for AI art” by Tim Brinkhof was published on 12/09/2024 by bigthink.com
Leave a Reply