Neural networks that imitate the workings of the human brain now often generate art, power computer vision, and drive many more applications. Now a neural network microchip from China that uses photons instead of electrons, dubbed Taichi, can run AI tasks as well as its electronic counterparts with a thousandth as much energy, according to a new study.
AI typically relies on artificial neural networks in applications such as analyzing medical scans and generating images. In these systems, circuit components called neurons—analogous to neurons in the human brain—are fed data and cooperate to solve a problem, such as recognizing faces. Neural nets are dubbed “deep“ if they possess multiple layers of these neurons.
“Optical neural networks are no longer toy models. They can now be applied in real-world tasks.” —Lu Fang, Tsinghua University, Beijing
As neural networks grow in size and power, they are becoming more energy hungry when run on conventional electronics. For instance, to train its state-of-the-art neural network GPT-3, a 2022 Nature study suggested OpenAI spent US $4.6 million to run 9,200 GPUs for two weeks.
The drawbacks of electronic computing have led some researchers to investigate optical computing as a promising foundation for next-generation AI. This photonic approach uses light to perform computations more quickly and with less power than an electronic counterpart.
Now scientists at Tsinghua University in Beijing and the Beijing National Research Center for Information Science and Technology have developed Taichi, a photonic microchip that can perform as well as electronic devices on advanced AI tasks while proving far more energy efficient.
“Optical neural networks are no longer toy models,” says Lu Fang, an associate professor of electronic engineering at Tsinghua University. “They can now be applied in real-world tasks.”
How does an optical neural net work?
Two strategies for developing optical neural networks either scatter light in specific patterns within the microchips, or get light waves to interfere with each other in precise ways inside the devices. When input in the form of light flows into these optical neural networks, the output light encodes data from the complex operations performed within these devices.
Both photonic computing approaches have significant advantages and disadvantages, Fang explains. For instance, optical neural networks that rely on scattering, or diffraction, can pack many neurons close together and consume virtually no energy. Diffraction-based neural nets rely on the scattering of light beams as they pass through optical layers that represent the network’s operations. One drawback of diffraction-based neural nets, however, is that they cannot be reconfigured. Each string of operations can essentially only be used for one specific task.
Taichi boasts 13.96 million parameters.
In contrast, optical neural networks that depend on interference can readily be reconfigured….
Read full article: AI Chip Trims Energy Budget Back by 99+ Percent
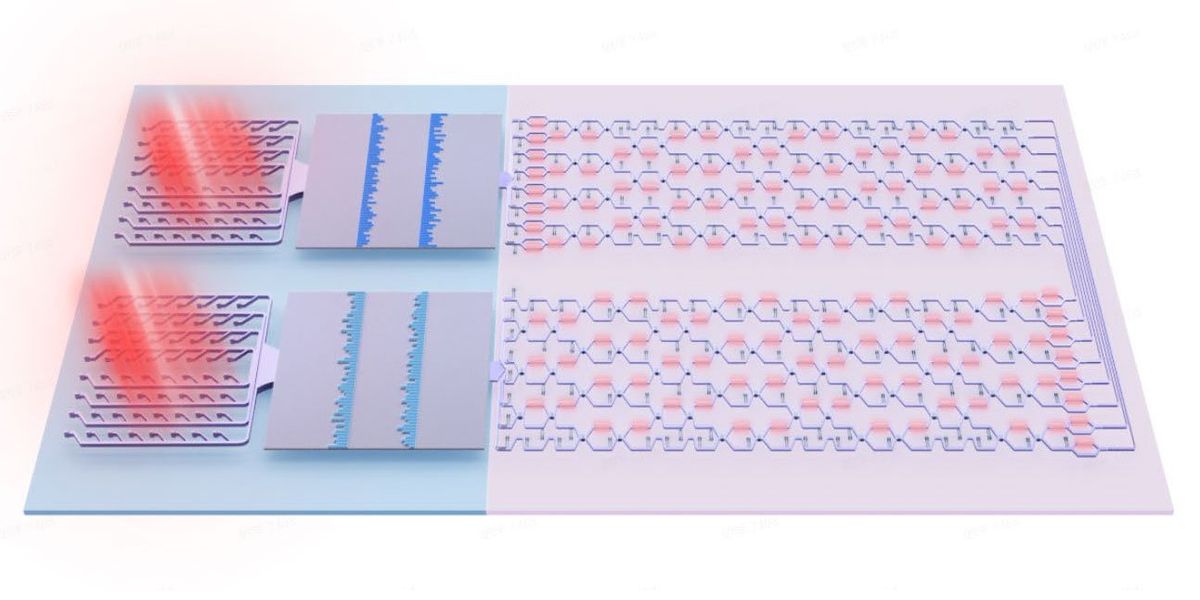
The post “AI Chip Trims Energy Budget Back by 99+ Percent” by Charles Q. Choi was published on 04/12/2024 by spectrum.ieee.org