An advanced algorithm that has been developed by Google DeepMind has gone some way to cracking one of the biggest unsolved mysteries in biology. AlphaFold aims to predict the 3D structures of proteins from the “instruction code” in their building blocks. The latest upgrade has recently been released. The latest upgrade has recently been released.
Proteins are essential parts of living organisms and take part in virtually every process in cells. But their shapes are often complex, and they are difficult to visualise. So being able to predict their 3D structures offers windows into the processes inside living things, including humans.
This provides new opportunities for creating drugs to treat disease. This in turn opens up new possibilities in what is called molecular medicine. This is where scientists strive to identify the causes of disease at the molecular scale and also develop treatments to correct them at the molecular level.
The first version of DeepMind’s AI tool was unveiled in 2018. The latest iteration, released this year, is AlphaFold3. A worldwide competition to evaluate new ways of predicting the structures of proteins, the Critical Assessment of Structure Prediction (Casp) has been held biannually since 1994 In 2020, the Casp competition got to test AlphaFold2 and was very impressed. Since then, researchers eagerly anticipate each new incarnation of the algorithm.
However, as a masters student I was once reprimanded for using AlphaFold2 in some of my coursework. This was because it was deemed only a predictive tool. In other words, how could anyone know whether what was predicted matched the real-life protein without experimental verification?
This is a legitimate point. The area of experimental molecular biology has undergone its own revolution in the past decade with strong advances in a microscope technique called cryo-electron microscopy (cryo-EM), which uses frozen samples and gentle electron beams to capture the structures of biomolecules in high resolution.
The advantage of AI tools such as AlphaFold is that it can elucidate protein structures much faster (in a matter of minutes) at almost no cost. Results are more readily available and accessible globally online. They can also predict the structure of proteins that are notoriously difficult to experimentally verify, such as membrane proteins.
However, AlphaFold2 was not designed to address something called the quaternary structure of proteins, where multiple protein subunits form a larger protein. This involves a dynamic visualisation of how different units of the protein molecule are folded. And some researchers reported that it sometimes appeared to have difficulty predicting structural elements of proteins known as coils.
Halfpoint / Shutterstock
When my professor contacted me in May to relay the news that AlphaFold3 had been released, my first question was about its ability to predict quaternary structures. Had it succeeded? Were we now able to take the massive leap towards predicting a complete structure? Early reports suggest the answers to those questions are positive.
Experimental methods are slower. And when they are able to capture the 3D structure of molecules, it is more akin to looking at a statue –- a snapshot of the protein – rather than seeing how it moves and interacts to carry out actions in the body. In other words, we want a movie, rather than a photo.
Experimental methods have also traditionally struggled with membrane proteins – key molecules that are attached to or are associated with the membranes of cells. These are often crucial in understanding and treating many of the worst diseases.
Here is where AlphaFold3 could truly change the landscape. If it is successful at predicting quaternary structures at a level equal to or greater than experimental methods such as crystallography, cryo-EM and others, and it can visualise membrane proteins better than the competition, then we will indeed have a gigantic leap forwards in our race towards true molecular medicine.
AlphaFold3 can only be accessed from a DeepMind server, but it is easy to use. Researchers can get their results in minutes simply from the sequence. The other promise of AlphaFold3 is further disruption. DeepMind is not alone in its ambitions to master the problem of protein folding. As the next Casp competition approaches there are others looking to win the race. For example, Liam McGuffin and his team at the University of Reading are making gains in quality assessment and predicting the stoichiometry of protein complexes. Stoichiometry refers to the proportions in which elements or chemical compounds react with one another.
Not all scientists in this area are chasing the goal in the same way. Others are trying to solve similar challenges in terms of the quality of the 3D models or specific barriers such as those presented by membrane proteins. The competition has been marvellous for progress in this field.
However, experimental methods are not going away anytime soon, and nor should they. The progress of cryo-EM is laudable, and X-ray crystallography still gives us the finest resolution on biomolecules. The European XFEL laser in Germany could be the next breakthrough. These technologies will only continue to improve.
My biggest question as we survey this new field is whether our human instinct to relent until we have absolute proof will fold with AlphaFold. If this new technology is able to give results comparable to, or greater than, experimental verification, will we be prepared to accept it? If we can, its speed and accuracy could have a major effect on areas such as drug development.
For the first time, with AlphaFold3, we may have cleared the most significant hurdle in the protein prediction revolution. What will we make of this new world? And what medicine can we make with it?
The post “An AI tool for predicting protein shapes could be transformative for medicine, but it challenges science’s need for proof” by Sam McKee, Tutor and researcher in Philosophy of Science, Manchester Metropolitan University was published on 05/31/2024 by theconversation.com
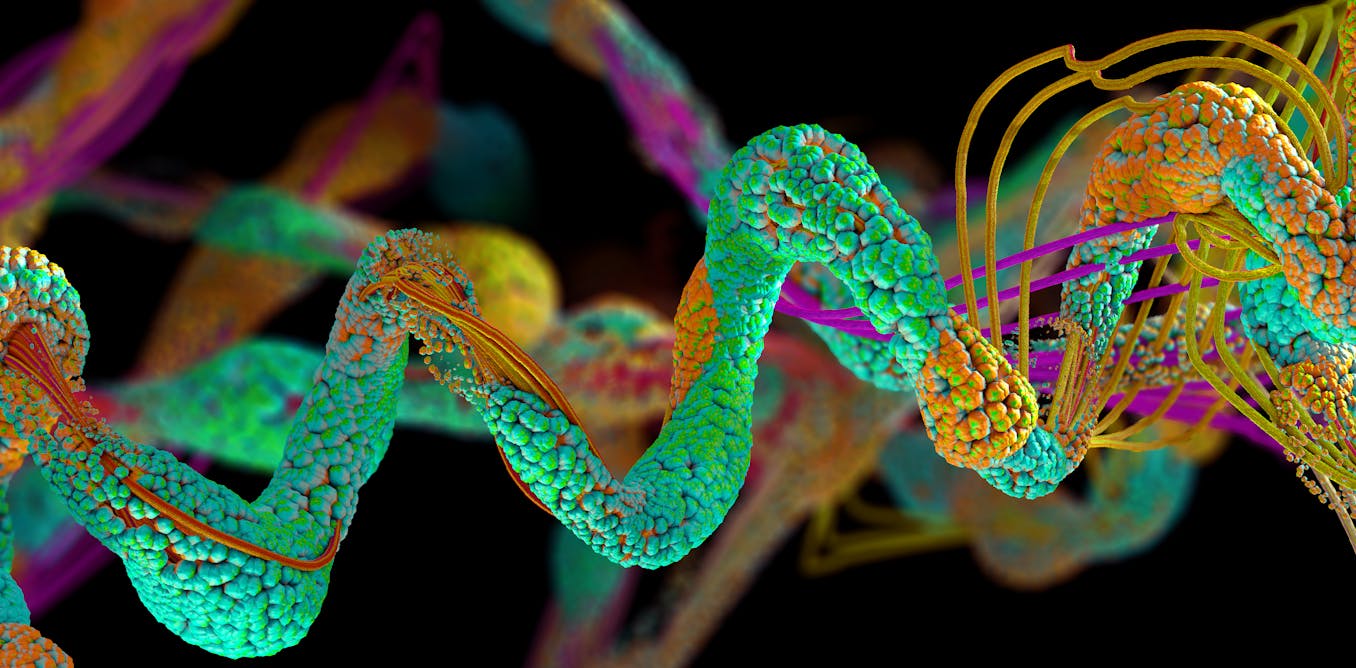
The post “An AI Tool with the Potential to Revolutionize Medicine Faces Scientific Skepticism – GretAi News” by GretAi was published on 05/31/2024 by news.gretai.com